Has AI avoided problems in the VC industry?
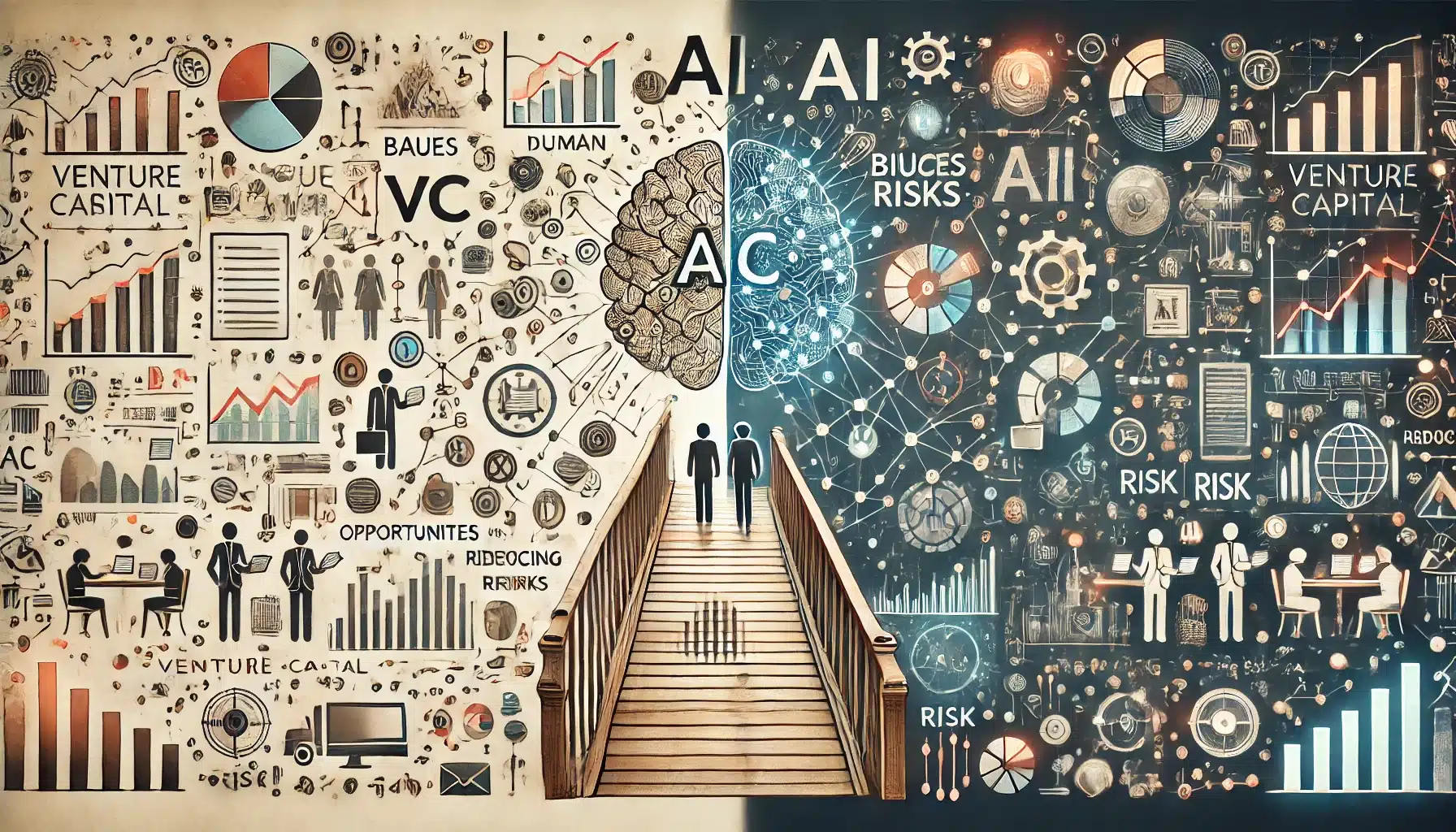
Artificial Intelligence (AI) is a branch of computer science focused on creating systems and machines that can perform tasks typically requiring human intelligence. These tasks include learning, problem-solving, decision-making, language understanding, and visual perception. AI systems use algorithms and data to analyze patterns, make predictions, and adapt to new information, often mimicking human cognitive processes. AI is widely used in various fields, including healthcare, finance, transportation, and entertainment, transforming how we interact with technology and solve complex problems.
The venture capital (VC) industry focuses on funding and supporting early-stage or high-growth startups and businesses with strong potential. Venture capital firms invest in these companies in exchange for equity, aiming to earn substantial returns if the businesses succeed. VC funding often comes in stages, such as seed, Series A, B, and beyond, as startups grow and scale. The industry plays a crucial role in fostering innovation by providing the financial resources and strategic guidance needed for emerging businesses to develop and thrive.
AI has brought significant innovations to the venture capital (VC) industry, addressing some of its traditional challenges but not entirely avoiding the problems inherent in this complex field. The VC industry, which is driven by high-risk investments in startups and early-stage companies, has long struggled with issues such as biased decision-making, inefficiencies in sourcing promising ventures, and the difficulty of predicting which businesses will thrive in uncertain markets. AI offers tools that can alleviate these challenges by providing data-driven insights, automating labor-intensive tasks, and enhancing the decision-making process.
One area where AI has shown promise is in deal sourcing. Traditionally, venture capitalists relied on personal networks and intuition to identify investment opportunities, a process that could overlook valuable prospects outside their immediate circles. AI-driven platforms can analyze large datasets from diverse sources, including social media, business registries, and market trends, to identify startups with high growth potential. This capability expands the pool of potential investments, allowing VCs to discover opportunities they might otherwise miss.
AI also enhances the evaluation of startups by analyzing key performance indicators, market data, and financial records to predict a company’s likelihood of success. Machine learning models can identify patterns and trends in historical data, offering insights into what factors correlate with successful outcomes. This helps venture capitalists make more informed decisions, potentially reducing the high failure rate that characterizes startup investments.
However, despite these advancements, AI has not completely solved the VC industry’s problems. One persistent issue is bias, which can be embedded in the data AI systems use to make predictions. If historical investment patterns reflect biases—such as favoring certain demographics, industries, or regions—AI models can inadvertently perpetuate or even amplify these disparities. This means that while AI has the potential to bring objectivity, it requires careful oversight to ensure that the data it relies on is equitable and representative.
Another limitation of AI in venture capital is its inability to fully capture the human and relational aspects of investing. Building trust, assessing a team’s chemistry, and understanding the vision and passion of founders are crucial elements of successful venture investing that cannot be quantified or automated. While AI can analyze past performance and market conditions, it cannot replace the nuanced judgment and intuition that come from human experience and interaction.
Moreover, the effectiveness of AI in venture capital depends heavily on the quality and quantity of data available. Startups, especially those in their early stages, often lack extensive data, making it challenging for AI to provide accurate assessments. In such cases, human expertise remains indispensable.
In essence, AI has become a valuable tool in the venture capital industry, helping to streamline processes, reduce inefficiencies, and provide data-driven insights. However, it has not entirely avoided the industry’s inherent challenges. Instead, it works best as a complement to traditional methods, augmenting human decision-making while requiring oversight to address its limitations. The integration of AI into venture capital reflects a broader shift toward combining technology with human expertise to navigate the complexities of high-risk, high-reward investing.
The intersection of artificial intelligence (AI) and venture capital (VC) presents both challenges and opportunities as the industry integrates these technologies into its decision-making processes. Some of the key challenges and their potential solutions include:
Data quality and availability: Startups often lack the extensive historical data needed for AI to make accurate predictions, especially in their early stages. AI systems rely on robust datasets to identify patterns and generate insights, but the limited and variable nature of startup data can hinder their effectiveness.
Solution: Combining AI with human expertise can address this limitation. Venture capitalists can use AI to analyze broader market trends, comparable company data, and other contextual information, while relying on their experience and intuition to evaluate startups with minimal data.
Bias in AI algorithms: AI systems trained on historical investment data may inadvertently reflect and perpetuate biases, such as favoring startups founded by certain demographics or located in specific regions. This bias undermines diversity and the discovery of innovative but unconventional ventures.
Solution: Implementing bias-mitigation techniques, such as diversifying training datasets and auditing algorithms for fairness, can reduce the risk of biased outcomes. Additionally, incorporating inclusive criteria into AI models can help identify opportunities outside traditional investment patterns.
Over-reliance on AI outputs: The perceived objectivity of AI can lead to over-reliance on its recommendations, potentially sidelining human judgment. Venture capital involves qualitative factors, such as team dynamics, vision, and adaptability, that AI cannot fully assess.
Solution: AI should be treated as an advisory tool rather than a decision-maker. VCs can use AI to support their evaluations and cross-check insights while ensuring that final decisions incorporate human intuition and qualitative assessments.
Dynamic market conditions: Startups often operate in rapidly evolving industries where market conditions can change unpredictably. AI models trained on historical data may struggle to adapt to these changes in real-time.
Solution: Continuous retraining and updating of AI models with fresh data can help improve their adaptability. Pairing AI insights with real-time market analysis by human experts ensures a more comprehensive understanding of evolving conditions.
Trust and transparency: Many investors and founders may be skeptical of AI’s role in VC due to its perceived “black box” nature. The lack of clarity in how AI models generate recommendations can hinder trust and adoption.
Solution: Enhancing the interpretability and explainability of AI models can build trust among stakeholders. By clearly communicating how recommendations are made and the factors influencing them, VCs can create confidence in AI-driven processes.
Scalability of insights: While AI can process large volumes of data, it may struggle to provide tailored insights for niche or highly innovative startups that defy standard metrics and patterns.
Solution: Combining AI-driven analytics with specialized human expertise allows for more nuanced evaluations. VCs can use AI to handle large-scale data processing while relying on sector-specific knowledge for unique cases.
By addressing these challenges, AI can become an integral part of the VC industry, enhancing efficiency, reducing risk, and uncovering new opportunities while preserving the human elements critical to successful investing.

Staff
Writer